Unlocking Opportunities: Utilizing Agriculture Datasets for Machine Learning
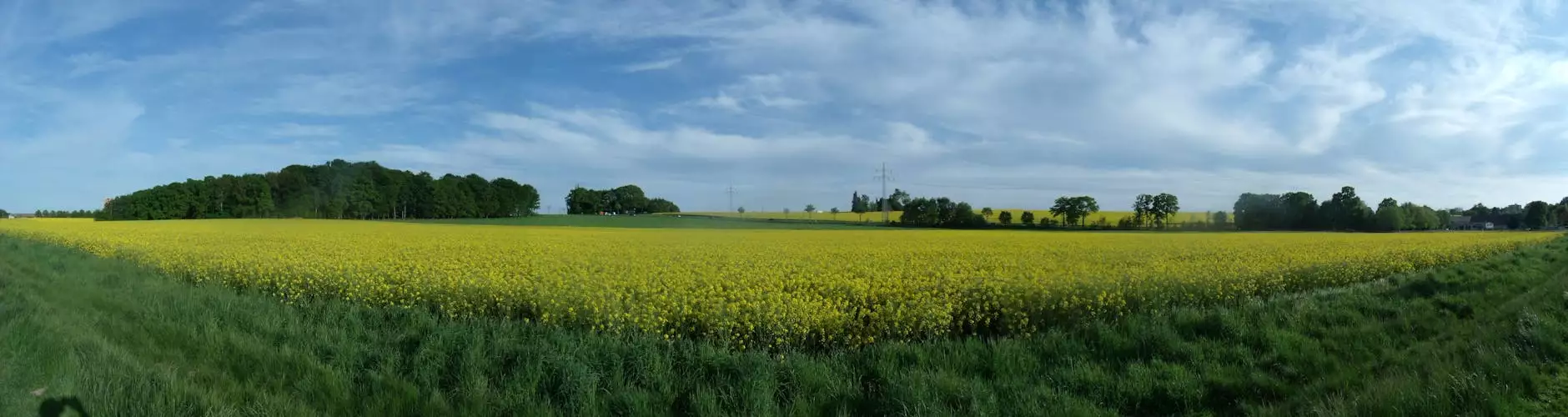
In the 21st century, the fusion of technology and agriculture has birthed a new era of possibilities. With the emergence of machine learning (ML) and artificial intelligence (AI), the agricultural landscape can now leverage data analysis to enhance productivity, sustainability, and efficiency. One of the key components in this transformation is the use of agriculture datasets for machine learning. This article delves into the significance, applications, and benefits of these datasets while exploring how they can revolutionize the farming industry.
Understanding Agriculture Datasets
Agriculture datasets consist of a variety of data points that are collected from farming activities. These datasets can be sourced from multiple channels:
- Remote Sensing: Satellite imagery provides insights into crop health, soil moisture, and weather conditions.
- IoT Devices: Smart sensors collect data on soil temperature, humidity, and nutrient levels.
- Historical Crop Data: Records of past crop yields, planting practices, and climatic conditions.
- Market Data: Information on crop prices, demand forecasts, and agricultural trends.
The Role of Machine Learning in Agriculture
Machine Learning, a subset of AI, empowers systems to learn from data, identify patterns, and make predictions without being explicitly programmed. In agriculture, this technology has found numerous applications:
1. Precision Agriculture
Precision agriculture utilizes agriculture datasets to make informed decisions on resource allocation. For example, by analyzing soil data, farmers can determine the best locations for planting, ensuring optimal yield while minimizing waste. This targeted approach helps in:
- Reducing input costs (fertilizers, water, pesticides).
- Enhancing crop quality and yield.
- Promoting sustainable farming practices.
2. Predictive Analytics
Leveraging historical data, machine learning algorithms can forecast future trends, helping farmers make proactive decisions. For instance, by analyzing weather data alongside historical yields, farmers can predict potential harvest sizes and adjust their planting strategies accordingly.
3. Pest and Disease Detection
With the help of image recognition technologies, machine learning models can analyze images from crops to detect signs of pest infestations or diseases early. This timely intervention can save large portions of crops and reduce the need for harmful pesticides.
4. Supply Chain Optimization
Machine learning can streamline the supply chain by predicting demand, optimizing logistics, and minimizing waste. By analyzing market data, farmers can decide the optimal time to harvest and market their products.
Benefits of Using Agriculture Datasets for Machine Learning
The integration of agriculture datasets with machine learning technologies brings about numerous benefits:
- Increased Efficiency: Automation of routine tasks and optimization of resource usage lead to lower operational costs.
- Higher Yields: Data-driven farming practices empower farmers to make informed choices, leading to improved crop yields.
- Sustainability: By using resources more efficiently and responsibly, farmers can reduce their environmental impact and promote sustainable agriculture.
- Enhanced Risk Management: Predictive models help in identifying potential risks, allowing farmers to take corrective action before losses occur.
Challenges in Data Utilization
While the advantages of utilizing agriculture datasets for machine learning are clear, there are challenges that must be addressed:
1. Data Quality and Accessibility
The reliability of insights drawn from machine learning models heavily depends on the quality and completeness of the datasets. Many datasets may be incomplete or noisy, leading to inaccurate predictions. Making high-quality, accessible data available is crucial.
2. Technical Expertise
The adoption of machine learning technologies requires a certain level of technical expertise. Farmers might need training or assistance in interpreting data effectively and implementing ML-algorithms in their operations.
3. Ethical and Privacy Concerns
The collection and use of data raise ethical questions, particularly concerning privacy. It is essential to establish clear guidelines to ensure data is used responsibly and that the privacy of individuals and organizations is protected.
Real-World Applications of Agriculture Datasets
Several organizations and farms worldwide have already begun harnessing the power of agriculture datasets and machine learning.
1. The Climate Corporation
The Climate Corporation utilizes a vast amount of data to provide farmers with insights on weather patterns and soil conditions, helping them make smarter decisions.
2. IBM Watson Decision Platform for Agriculture
IBM’s platform combines AI, weather data, and IoT data to assist farmers in increasing crop yield while maintaining sustainability.
3. Climate FieldView
This platform offers farmers a comprehensive view of their operations by integrating various data sources, allowing for data-driven decisions.
Future Perspectives in Agricultural Data Science
The future of agriculture is undoubtedly bright, with ongoing advancements in technology paving the way for further innovations. The rise of agriculture datasets for machine learning not only promises to enhance efficiency but also to support ecological balance and sustainability.
1. Integration with Blockchain Technology
Blockchain technology can enhance the transparency and traceability of food supply chains, and when combined with ML, can transform how data is utilized in agriculture.
2. Enhanced Data Sharing Platforms
The development of platforms that facilitate the sharing of agricultural data among stakeholders can enhance collaborative efforts and innovations in farming practices.
Conclusion
Investing in agriculture datasets for machine learning is not just a trend; it is an essential step towards future-ready farming. As the agriculture industry continues to evolve, those who effectively utilize data-driven insights will thrive in an increasingly competitive market. By embracing technology and innovation, farmers can unlock limitless opportunities, driving not just their success but also contributing to global food security.
As we look to the horizon, the fusion of agriculture and technology emerges as a beacon of promise. The journey of innovation has just begun, and by leveraging the power of data, we can cultivate a more productive, sustainable, and prosperous agricultural future.
agriculture dataset for machine learning